2. Labeling Hair Follicles for AI Training: A Key Step for Accurate Predictions in Hair Health Detection
- zezeintel
- Feb 16
- 5 min read
Updated: Feb 17
In the process of leveraging AI for hair health detection, one of the most critical steps is the labeling of data used to train the machine learning model. For AI models, particularly deep learning models such as Yolov7, to generate accurate predictions, they must be trained on well-labeled data that is properly tagged and annotated. Specifically, in hair health detection, the task involves labeling hair follicle openings based on key indicators like hair density and hair thickness. This data labeling process directly affects the accuracy of the model's predictions, making it a crucial component of the training pipeline.
This article will explore in detail how hair follicle openings are labeled for AI training, the importance of this process, and its role in developing a reliable AI model capable of assessing hair health.
Why Labeling Hair Follicles is Crucial
Artificial intelligence, especially in computer vision tasks, learns by analyzing extensive datasets labeled with precise and meaningful information. When training a model such as Yolov7, designed for object detection in images, labels serve as the "ground truth" that the model utilizes for learning and making predictions. In the context of hair detection, the model must identify hair follicle openings as distinct targets for training.
Each follicle opening signifies a critical area on the scalp, providing valuable insights into hair density and thickness. By accurately labeling these follicles, the AI model can learn to discern the specific characteristics of both healthy and unhealthy hair. This labeled data guides the Yolov7 model during its training phase, ultimately enhancing its ability to recognize these hair follicles in new, unseen images.
The Process of Labeling Hair Follicle Openings
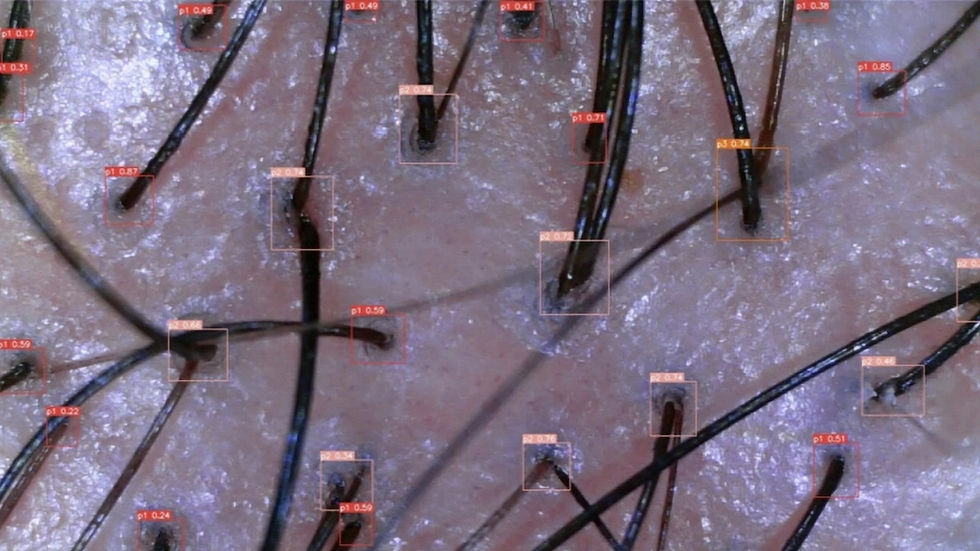
Labeling hair follicle openings for training Yolov7 involves a few key steps. This process is vital because it ensures that the AI model is exposed to well-annotated images, which allow it to learn the exact features necessary for accurate hair health detection.
Identifying Hair Follicle Openings
Hair follicle openings are natural openings in the scalp where hair strands grow. These openings vary in size, shape, and number of hairs per follicle. The first step in labeling is to identify these openings within an image. The model needs to distinguish between a hair follicle opening and the surrounding scalp, hair, or other structures in the image. This step requires accurate image segmentation to ensure the follicle openings are correctly marked and isolated from other irrelevant features.
Categorizing Hair Follicle Openings by Density and Thickness
Once hair follicle openings are identified, the next task is to categorize them based on hair density and hair thickness. Hair density refers to the number of hair follicles per square centimeter of scalp, while hair thickness measures the diameter of individual hair strands.
Single Hair Follicle (p1): This label is used when a hair follicle opening contains only one hair. This is typically seen in areas with lower density, such as in early stages of hair thinning or in certain parts of the scalp.
Two Hairs per Follicle (p2): This category is used when a follicle opening contains two hairs. It is common in areas with moderate hair density.
Multiple Hairs per Follicle (p3): This label applies when a follicle opening has three or more hairs, indicating a healthy and dense scalp. The label helps the AI model understand areas with higher hair growth.
This categorization enables the Yolov7 model to learn the patterns of hair distribution across the scalp, providing it with valuable information about hair health.
Focus on Hair Roots for Labeling
To further enhance the model’s accuracy, the labeling process focuses on the root of the hair rather than just the hair shaft. This is an important distinction because the hair root is tightly connected to the hair follicle and is distinguishable, making it an ideal target for detection. By labeling the hair root at the follicle opening, the AI model can effectively detect the presence of hair at its growth point, which is crucial for assessing overall hair health and density.
Labeling only the root ensures that the AI model focuses on the core area of hair growth and avoids unnecessary complications that may arise from labeling other parts of the hair shaft. This attention to detail enhances the model's ability to distinguish between different hair types and conditions.
Ensuring Consistency Across the Dataset
Consistency is key when labeling data for AI training. It’s essential that all images in the dataset are labeled according to the same rules and standards. This helps the AI model learn uniformly, without biases or inconsistencies in the labeling process.
For example, if one image has a follicle opening labeled as "p1" (single hair) but is categorized differently in another image, the model will become confused, which can lead to inaccurate predictions. Therefore, it’s critical to ensure that the labeling process follows a well-defined set of guidelines that remain consistent across all training images.
Using High-Quality Image Data
The quality of the images used for labeling also plays a significant role in the training process. High-quality images with clear, well-lit views of the scalp and hair follicles are necessary for accurate labeling. Low-resolution or blurry images can result in poorly labeled data, which in turn can affect the performance of the AI model. High-quality images enable the AI to detect fine details, such as the subtle differences in hair follicle openings and hair thickness, leading to better model accuracy.
The Role of Labeled Data in Yolov7’s Training
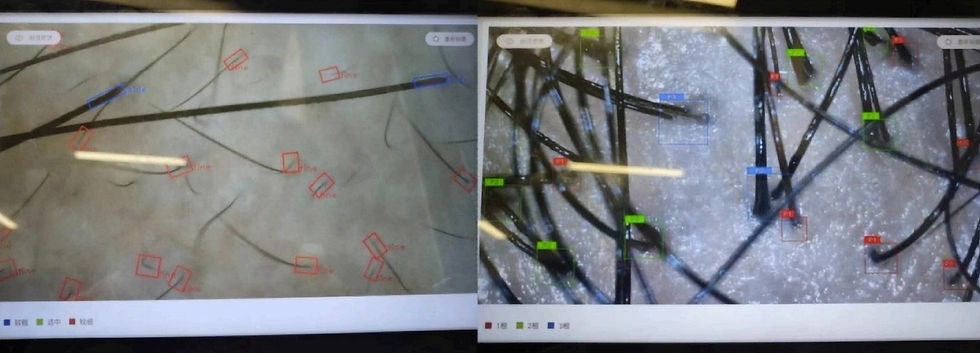
Once the hair follicle openings are labeled with the appropriate categories (p1, p2, p3), this labeled data is used to train the Yolov7 model. Yolov7 utilizes these labels to perform supervised learning, where the model is shown the labeled images and learns to associate the visual features in the images with the corresponding labels.
During training, the Yolov7 model learns to predict the location of the follicle openings and their respective labels. It adjusts its internal parameters to minimize errors between its predictions and the labeled ground truth. The more accurately the data is labeled, the better the model can learn, leading to higher performance when it is deployed for real-world applications.
Labeling as the Foundation for Accurate AI Predictions
In conclusion, the process of labeling hair follicle openings for AI training is essential for developing an effective hair detection model. By categorizing follicles according to their density and thickness, focusing on the hair root, ensuring consistency, and utilizing high-quality images, businesses can develop a highly accurate Yolov7 model capable of reliably detecting hair health conditions.
As the accuracy of the training data improves, the AI models' ability to predict and assess hair health in real time also enhances. The more precise the labels, the more accurate the AI model will be, making this step a crucial component of the AI training pipeline.
Comments